Models and Forecasting: Illuminating the Path to Informed Decision-Making
Post Date:
Business Coding Data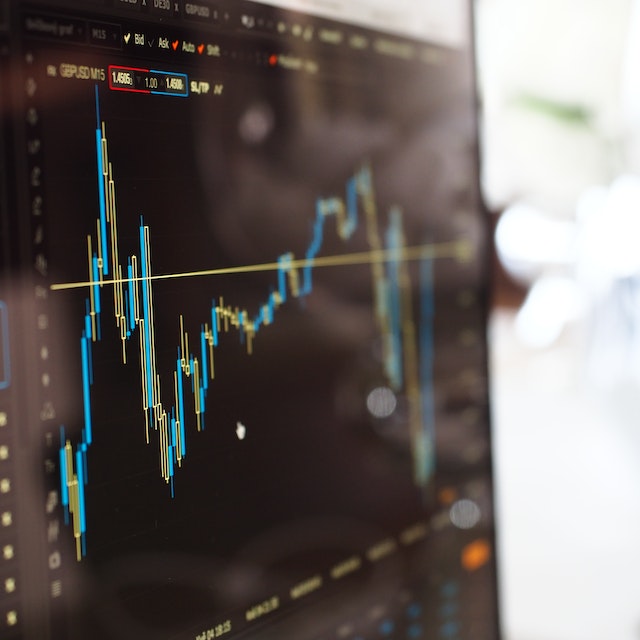
Forecasting is a crucial aspect of decision-making across various domains, from business and finance to climate science and epidemiology. Numerous modelling techniques have been developed and refined to make informed predictions about future events. These models can be broadly categorized into several types, each tailored to specific forecasting scenarios.
Time Series Models: Time series models are used when dealing with data points collected over time, such as stock prices, temperature readings, or sales figures. These models assume that the future values of a variable depend on its past importance. Standard time series models include Autoregressive Integrated Moving Average (ARIMA) and Seasonal Decomposition of Time Series (STL).
Regression Models: Regression models are versatile tools for forecasting that establish relationships between a dependent variable and one or more independent variables. Simple linear regression is used when there is a linear relationship, while multiple regression incorporates multiple predictors. Polynomial regression accommodates nonlinear relationships by including polynomial terms.
Exponential Smoothing Models: Exponential smoothing models are suitable for time series data characterized by seasonal or trend patterns. These models assign weights to past observations, with exponentially decreasing weights as observations move further into the past. Models like Exponential Smoothing (ETS) and Holt-Winters capture different aspects of time series data.
Machine Learning Models: Machine learning has revolutionized forecasting by leveraging complex algorithms and patterns in data. Decision trees, random forests, and gradient boosting are tree-based models that excel in capturing nonlinear relationships. Support Vector Machines (SVM), neural networks, and Long Short-Term Memory (LSTM) networks are used for more complex and high-dimensional forecasting tasks.
ARIMA Models: Autoregressive Integrated Moving Average (ARIMA) models are specifically designed for time series forecasting. They combine autoregressive (AR) and moving average (MA) components with differencing to make time series stationary. ARIMA models are effective in capturing trends and seasonality.
Seasonal Models: Seasonal models are tailored to handle data exhibiting regular seasonal patterns. Seasonal decomposition of time series (STL) is a popular method for decomposing a time series into its seasonal, trend, and residual components, allowing for more accurate forecasting.
Causal Models: Causal models, or econometric models, are used to understand cause-and-effect relationships between variables. These models incorporate factors that influence the variable being forecasted. Examples include autoregressive integrated moving averages with exogenous variables (ARIMAX) and vector autoregression (VAR).
Hybrid Models: Hybrid models combine multiple forecasting methods to improve accuracy. One common approach is combining ARIMA and machine learning models, leveraging the strengths of both systems. Hybrid models can be particularly effective when dealing with complex and noisy data.
Qualitative Models: Qualitative models are employed when historical data is scarce or unavailable. These models rely on expert judgment, surveys, or market research to make forecasts. Delphi method, market research-based forecasting, and expert opinion are examples of qualitative forecasting techniques.
Monte Carlo Simulation: Monte Carlo simulation is a probabilistic forecasting technique for uncertainty and variability. It involves running multiple simulations with random input values to generate a distribution of possible outcomes. This approach is instrumental in risk analysis and project management.
Choosing the appropriate forecasting model depends on the nature of the data, the forecasting horizon, the availability of historical data, and the specific objectives of the forecasting task. A well-chosen model can provide valuable insights and support informed decision-making, enabling organizations and researchers to plan more confidently.
Last Update: Sept. 11, 2023, 12:13 p.m.